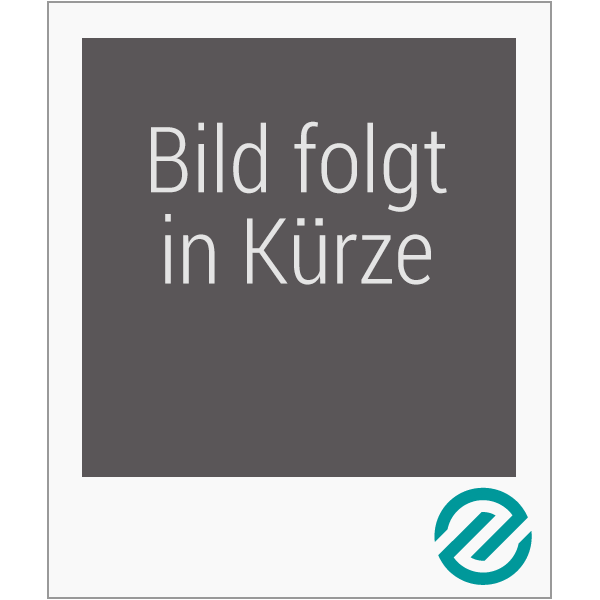
Multitemporal Earth Observation Image Analysis
Remote Sensing Image Sequences
Herausgeber: Mallet, Clément; Chehata, Nesrine
Versandkostenfrei!
Versandfertig in über 4 Wochen
152,99 €
inkl. MwSt.
PAYBACK Punkte
76 °P sammeln!
Earth observation has witnessed a unique paradigm change in the last decade with a diverse and ever-growing number of data sources. Among them, time series of remote sensing images has proven to be invaluable for numerous environmental and climate studies. Multitemporal Earth Observation Image Analysis provides illustrations of recent methodological advances in data processing and information extraction from imagery, with an emphasis on the temporal dimension uncovered either by recent satellite constellations (in particular the Sentinels from the European Copernicus programme) or archival aer...
Earth observation has witnessed a unique paradigm change in the last decade with a diverse and ever-growing number of data sources. Among them, time series of remote sensing images has proven to be invaluable for numerous environmental and climate studies. Multitemporal Earth Observation Image Analysis provides illustrations of recent methodological advances in data processing and information extraction from imagery, with an emphasis on the temporal dimension uncovered either by recent satellite constellations (in particular the Sentinels from the European Copernicus programme) or archival aerial images available in national archives. The book shows how complementary data sources can be efficiently used, how spatial and temporal information can be leveraged for biophysical parameter estimation, classification of land surfaces and object tracking, as well as how standard machine learning and state-of-the-art deep learning solutions can solve complex problems with real-world applications.