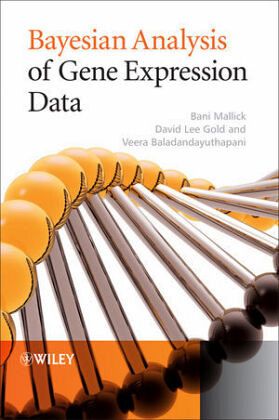
Bayesian Analysis of Gene Expression Data
PAYBACK Punkte
45 °P sammeln!
Providing an accessible overview of both Bayesian methods and gene expression, Bayesian Analysis of Gene Expression Data features numerous problems and solutions that emphasize methodology and application. Offering a clear introduction to the computational methods required for Bayesian statistics, the book provides an extensive review of Bayesian analysis and advanced topics for bioinformatics. A supplementary website including relevant software and further datasets round out the coverage in this text destined to become a vital resource for graduate students, medical consultants, and employees...
Providing an accessible overview of both Bayesian methods and gene expression, Bayesian Analysis of Gene Expression Data features numerous problems and solutions that emphasize methodology and application. Offering a clear introduction to the computational methods required for Bayesian statistics, the book provides an extensive review of Bayesian analysis and advanced topics for bioinformatics. A supplementary website including relevant software and further datasets round out the coverage in this text destined to become a vital resource for graduate students, medical consultants, and employees of private firms working with gene expression data. This book provides an introduction to both Bayesian methods and gene expression, accessible to people with backgrounds in either. The text is enhanced by the inclusion of numerous problems and solutions, designed with an emphasis on methodology and application. There is also a supplementary website including the relevant software and further datasets. The book provides an extensive review of Bayesian analysis and advanced topics for bioinformatics, including an advanced section that extensively details the necessary applications and offers an introduction to the computational methods required for Bayesian statistics.
The book is split into four parts,
Part I introducing the fundamentals of Bayesian statistics, both modeling and computation.
Part II builds on this, providing a more detailed introduction to the computational methods necessary for Bayesian statistics.
Part III presents an introduction to the basic biology needed to work with microarrays, written specifically to enable those readers with no experience in the laboratory to understand.
Part IV elaborates extensively on applications, including advanced topics for Bioinformatics research.
The book is split into four parts,
Part I introducing the fundamentals of Bayesian statistics, both modeling and computation.
Part II builds on this, providing a more detailed introduction to the computational methods necessary for Bayesian statistics.
Part III presents an introduction to the basic biology needed to work with microarrays, written specifically to enable those readers with no experience in the laboratory to understand.
Part IV elaborates extensively on applications, including advanced topics for Bioinformatics research.